High quality data requires ongoing effort to deliver reliable, timely, actionable e-commerce insights
Data and analytics play an ever-increasing role in decision making for product manufacturers, especially when it comes to planning strategies, and identifying and prioritizing actions to support the e-commerce channel.
The ability to readily measure and improve critical e-commerce performance indicators, such as Availability, Content, Price & Promotions, Search, and Ratings & Reviews is central to managing e-commerce success. With accurate and timely data brands can systematically identify gaps in product listings, user reviews, iMedia and content, to prioritize actions, and execute e-commerce perfect store performance improvements.
However, the growing dependence on data for everything from shipping and logistics planning, to customer service and e-commerce intelligence leaves brands open to problems associated with poor data quality. Bad data means less effective decision-making and an inability to plan and execute strategy. More subtly perhaps, poor data quality hurts employee morale, breeds organizational mistrust, and makes it more difficult to align the business.
Ongoing Data Quality
According to US analyst firm Gartner, the term “data quality” relates to the “processes and technologies for identifying, understanding and correcting flaws in data” to support effective business processes and decision-making. Ensuring data is always high quality requires ongoing effort. It’s not just a one-off activity. To make the very most of e-commerce data available, and maximize the value of the insights it delivers needs continuous quality management to protect and maintain data integrity across its entire lifecycle.
At eStoreMedia we strive to consistently maintain and improve the quality of the data at the core of our e-commerce analytics, so brands can always trust our insights to power positive, data-driven, decision making. The three key areas of focus for achieving the highest standards of data quality include the breadth of data collected and used in eStoreCheck, accurate product matching across online retailers, and a combination of automated and manual data quality checks.
More Data Means Better Quality
The difference between high-quality data and “bad” data might seem like a straightforward question. Is the data right or wrong, accurate or inaccurate? However, true data quality depends on more than simple accuracy, it also requires completeness.
If you only have a proportion of the relevant information such as tracking a limited number of known SKUs, no matter how correct the data its quality can’t be guaranteed. In e-commerce intelligence, a foundation based on full category data that includes Availability, Content, Price & Promotions, Search Performance, and Ratings & Reviews information on every SKU in a category is the starting point for delivering high quality data for accurate, timely, and robust insights.
Precise Product Matching
A key element of the insights brands expect from e-commerce analytics includes comparing listings, content and performance across rival online retailers in each market. Useful cross-retailer analysis needs accurate identification and matching of duplicate products, with or without universal identifiers such as UPCs, or GTINs.
When available such identifiers can provide reliable product matching, but they can’t be depended on 100% of the time. Some online retailers don’t publish UPCs on the product page, and many others such as Amazon use their own product identifiers in combination with UPCs. In such situations, relying on identifiers alone can lead to inaccurate product matching that may point to issues that don’t exist, undermining trust in the analytics process.
Achieving accurate product matches requires an aggregated approach. For example, eStoreMedia backs-up UPC matching with content analysis and image comparison to ensure matches are 100% accurate. Matching products in terms of their titles, features such as brand, color, and size, and comparing across different data sources for the same product, increases the accuracy of product matching for reliable cross retailer insights.
Automated & Manual Data Checks
Even with full category data and accurate product matching, maintaining high-quality data requires ongoing analysis and intervention. Retailers frequently change the layout of their categories and landing pages, which means collecting accurate e-commerce data is an ongoing battle that requires constant adaptation.
eStoreMedia has developed dozens of automated data quality checks, that are built into its data collection and analysis processes. Conflicting category product counts, high price differentials or missing data can be indicators of data quality issues that immediately trigger new retailer or category scans for example.
Starting from a foundation of full category data also helps with automating data quality analysis. It might seem counter intuitive that processing more data could lead to improved output. However, full category data means we can run more accurate statistical analyses to identify anomalies, and data quality problems, and fix them on the fly. If issues persist, the next line of defense for data quality maintenance will involve manual intervention to correct the problem.
Quality Data Drives Quality Decisions
To be successful online product manufactures need e-commerce data to make important decisions, protect their brands and drive sustainable online channel sales growth. But, data quality can never be taken for granted, it needs to be integral to the processes of collecting, updating, and managing data.
Register for a demonstration to find out more about how eStoreMedia ensures the quality of the data at the centre of its e-commerce analytics solutions.
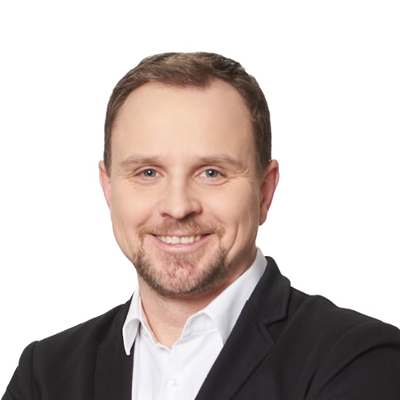